SapienzaNLP @ IJCAI 2020
3 papers accepted: from WSD to Vision!
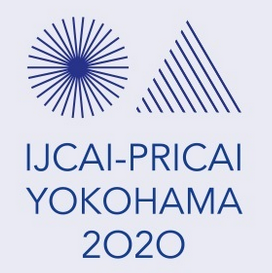
- EViLBERT: Learning Task-Agnostic Multimodal Sense Embeddings
- MuLaN: Multilingual Label propagatioN for Word Sense Disambiguation
- The Knowledge Acquisition Bottleneck Problem in Multilingual Word Sense Disambiguation
EViLBERT: Learning Task-Agnostic Multimodal Sense Embeddings
by Agostina Calabrese, Michele Bevilacqua and Roberto Navigli
The problem of grounding language in vision is increasingly attracting scholarly efforts. As of now, however, most of the approaches have been limited to word embeddings, which are not capable of handling polysemous words. This is mainly due to the limited coverage of the available semantically-annotated datasets, hence forcing research to rely on alternative technologies (i.e., image search engines). To address this issue, we introduce EViLBERT, an approach which is able to perform image classification over an open set of concepts, both concrete and non-concrete. Our approach is based on the recently introduced Vision-Language Pretraining (VLP) model, and builds upon a manually-annotated dataset of concept-image pairs. We use our technique to clean up the image-to-concept mapping that is provided within a multilingual knowledge base, resulting in over 258,000 images associated with 42,500 concepts. We show that our VLP-based model can be used to create multimodal sense embeddings starting from our automatically-created dataset. In turn, we also show that these multimodal embeddings improve the performance of a Word Sense Disambiguation architecture over a strong unimodal baseline.
MuLaN: Multilingual Label propagatioN for Word Sense Disambiguation
by Edoardo Barba, Luigi Procopio, Niccolò Campolungo, Tommaso Pasini and Roberto Navigli
The knowledge acquisition bottleneck strongly affects the creation of multilingual sense-annotated data, hence limiting the power of supervised systems when applied to multilingual Word Sense Disambiguation. In this paper, we propose a semi-supervised approach based upon a novel label propagation scheme, which, by jointly leveraging contextualized word embeddings and the multilingual information enclosed in a knowledge base, projects sense labels from a high-resource language, i.e., English, to lower-resourced ones. Backed by several experiments, we provide empirical evidence that our automatically created datasets are of a higher quality than those generated by other competitors and lead a supervised model to achieve state-of-the-art performances in all multilingual Word Sense Disambiguation tasks.
The Knowledge Acquisition Bottleneck Problem in Multilingual Word Sense Disambiguation
by Tommaso Pasini
Word Sense Disambiguation (WSD) is the task of identifying the meaning of a word in a given context. It lies at the base of Natural Language Processing as it provides semantic information for words. In the last decade, great strides have been made in this field and much effort has been devoted to mitigate the knowledge acquisition bottleneck problem, i.e., the problem of semantically annotating texts at a large scale and in different languages. This issue is ubiquitous in WSD as it hinders the creation of both multilingual knowledge bases and manually-curated training sets. In this work, we first introduce the reader to the task of WSD through a short historical digression and then take the stock of the advancements to alleviate the knowledge acquisition bottleneck problem. In that, we survey the literature on manual, semi-automatic and automatic approaches to create English and multilingual corpora tagged with sense annotations and present a clear overview over supervised models for WSD. Finally, we provide our view over the future directions that we foresee for the field.